As evaluators, we’ve already begun exploring how Artificial Intelligence can enhance the way we work. However, our recent panel at the European Evaluation Society (EES) conference highlighted a second, vital question:
How Do We Evaluate AI in Public Policy?
Artificial Intelligence (AI) has the power to revolutionise global development, transforming public policy and programming. But with this power comes a critical challenge: how do we evaluate AI systems to ensure they are effective, responsible, fair and have positive impacts on society?
The Opportunity and Challenge of AI
AI is an exciting tool with immense potential to enhance public services. Yet, it is new, largely untested, and fraught with unique risks, including biases in data and unintended societal impacts. It’s not enough to marvel at AI’s potential; we must ask tough questions:
- Does AI improve public services and resource allocation?
- Is the data AI uses unbiased, consensual, and transparent?
Evaluation is essential for holding AI accountable, understanding its societal impact and feeding insights back into program design. Without robust evaluation, AI risks being a shiny tool with limited value or, worse, causing harm.
Read more: promoting equitable, ethical AI use
What does this mean for evaluators?
With AI likely to feature more and more as part of public services, it raises a range of new considerations and responsibilities for evaluation, requiring us to grapple with additional questions:
- Fairness: does the AI treat all groups equitably, and how do we identify and address biases?
- Ethics: is the data safe, and does it respect privacy, consent, and transparency?
At the same time, traditional evaluation criteria remain essential and relevant to AI:
- Effectiveness: has the AI met its objectives, such as reducing dropout rates?
- Sustainability: can the system operate independently over the long term without external support?
- Impact: does the AI deliver meaningful improvements in societal outcomes?
The tools and frameworks we already use can be adapted to address these new challenges, providing a strong foundation for evaluating AI in public policy.
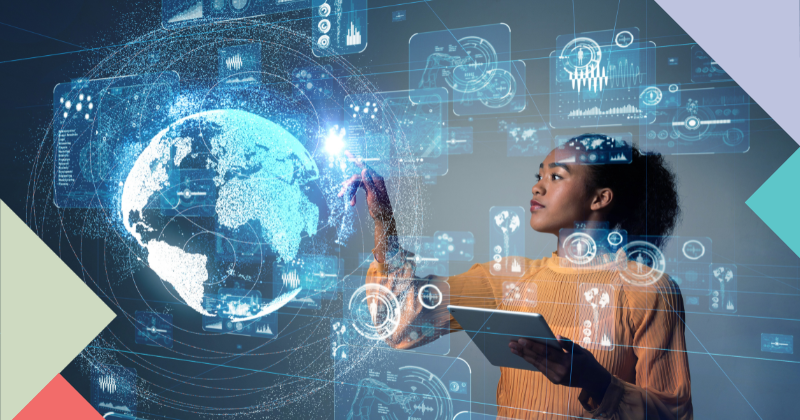
We must test and evidence emerging theories of AI use
As AI becomes more embedded in public programs, certain theories of its use are beginning to emerge and need rigorous testing:
- Efficiency Theory: AI can deliver services faster and with fewer resources.
- Personalization Theory: AI enables tailored, citizen-focused services, especially in health and education.
- Prediction Theory: AI’s ability to forecast and prevent issues (e.g., student dropouts) can save resources and alleviate suffering.
Building an evidence base around these theories will help guide future applications of AI, particularly in diverse contexts such as low-resource settings or in different cultures.
What new skills do evaluators need in an AI world?
Evaluators don’t need to become data scientists, but a foundational understanding of AI is crucial, including:
- basic knowledge of machine learning and statistical principles
- awareness of tools and techniques for mitigating bias and ensuring ethical AI
- access to specialised expertise when needed, particularly for evaluating complex algorithms
What does this look like in practice? Evaluating AI use in Mexico’s education sector
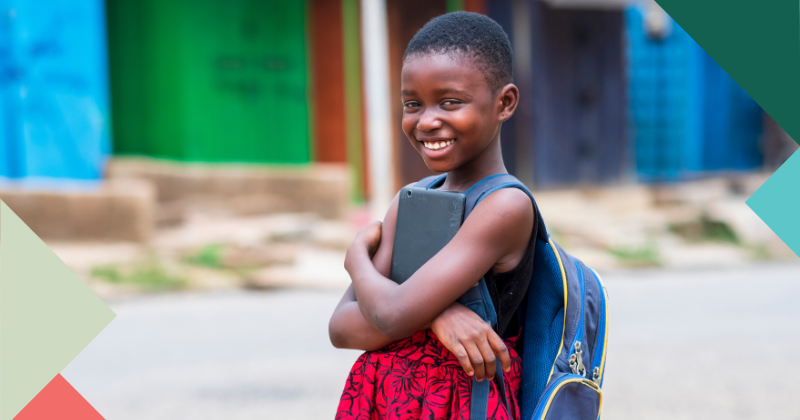
Partnering with the World Bank, the Ministry of Education in Guadalajara, Mexico, has launched an AI early warning system to combat school dropouts. This AI model analyses data on school-aged children, including educational assessments, family backgrounds, disciplinary history and counselling services to predict which students are at risk of dropping out – and enable targeted interventions.
To ensure the AI model was free from bias, we were given a grant by USAID to work with a consortium of partners to assess the system using IBM’s open-source program, AI Fairness 360.
By accessing data before and after processing by the algorithm, we revealed that the AI model was failing to identify one in four at-risk girls due to critical gender biases in the data. As such, our award-winning evaluation ensured that 4% more girls received the support needed to stay in school.
Building on this work, we have developed various guides and toolkits to assist policymakers and those designing AI models in the education sector.
Read more: USAID’s equitable AI challenge
It’s clear that AI is here to stay, with the potential to significantly accelerate solutions to global challenges. However, for this to happen, policymakers, implementers and evaluators must work together to ensure AI is used fairly and ethically.
To find out more about how we’re exploring the ways AI impacts evaluation, get in touch with Rob Lloyd.